Linear Digressions
Podcast készítő Ben Jaffe and Katie Malone
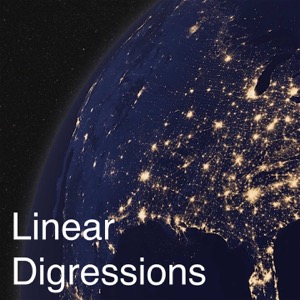
Kategóriák:
289 Epizód
-
Network effects re-release: when the power of a public health measure lies in widespread adoption
Közzétéve: 2020. 03. 15. -
Causal inference when you can't experiment: difference-in-differences and synthetic controls
Közzétéve: 2020. 03. 09. -
Better know a distribution: the Poisson distribution
Közzétéve: 2020. 03. 02. -
The Lottery Ticket Hypothesis
Közzétéve: 2020. 02. 23. -
Interesting technical issues prompted by GDPR and data privacy concerns
Közzétéve: 2020. 02. 17. -
Thinking of data science initiatives as innovation initiatives
Közzétéve: 2020. 02. 10. -
Building a curriculum for educating data scientists: Interview with Prof. Xiao-Li Meng
Közzétéve: 2020. 02. 02. -
Running experiments when there are network effects
Közzétéve: 2020. 01. 27. -
Zeroing in on what makes adversarial examples possible
Közzétéve: 2020. 01. 20. -
Unsupervised Dimensionality Reduction: UMAP vs t-SNE
Közzétéve: 2020. 01. 13. -
Data scientists: beware of simple metrics
Közzétéve: 2020. 01. 05. -
Communicating data science, from academia to industry
Közzétéve: 2019. 12. 30. -
Optimizing for the short-term vs. the long-term
Közzétéve: 2019. 12. 23. -
Interview with Prof. Andrew Lo, on using data science to inform complex business decisions
Közzétéve: 2019. 12. 16. -
Using machine learning to predict drug approvals
Közzétéve: 2019. 12. 08. -
Facial recognition, society, and the law
Közzétéve: 2019. 12. 02. -
Lessons learned from doing data science, at scale, in industry
Közzétéve: 2019. 11. 25. -
Varsity A/B Testing
Közzétéve: 2019. 11. 18. -
The Care and Feeding of Data Scientists: Growing Careers
Közzétéve: 2019. 11. 11. -
The Care and Feeding of Data Scientists: Recruiting and Hiring Data Scientists
Közzétéve: 2019. 11. 04.
In each episode, your hosts explore machine learning and data science through interesting (and often very unusual) applications.