#220 What, why, and where of friendly errors in Python
Python Bytes - Podcast készítő Michael Kennedy and Brian Okken - Hétfők
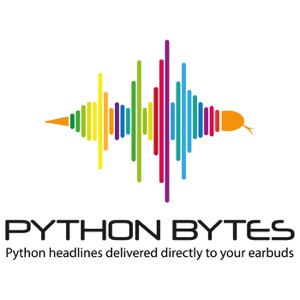
Kategóriák:
Sponsored by Datadog: pythonbytes.fm/datadog Special guest: Hannah Stepanek Watch on YouTube Michael #1: We Downloaded 10,000,000 Jupyter Notebooks From Github – This Is What We Learned by Alena Guzharina from JetBrains Used the hundreds of thousands of publicly accessible repos on GitHub to learn more about the current state of data science. I think it’s inspired by work showcased here on Talk Python. 2 years ago there were 1,230,000 Jupyter Notebooks published on GitHub. By October 2020 this number had grown 8 times, and we were able to download 9,720,000 notebooks. 8x growth. Despite the rapid growth in popularity of R and Julia in recent years, Python still remains the most commonly used language for writing code in Jupyter Notebooks by an enormous margin. Python 2 went from 53% → 11% in the last two years. Interesting graphs about package usage Not all notebooks are story telling with code: 50% of notebooks contain fewer than 4 Markdown cells and more than 66 code cells. Although there are some outliers, like notebooks with more than 25,000 code lines, 95% of the notebooks contain less than 465 lines of code. Brian #2: pytest-pythonpath plugin for adding to the PYTHONPATH from the pytests.ini file before tests run Mentioned briefly in episode 62 as a temporary stopgap until you set up a proper package install for your code. (cringing at my arrogance). Lots of projects are NOT packages. For example, applications. I’ve been working with more and more people to get started on testing and the first thing that often comes up is “My tests can’t see my code. Please fix.” Example proj/src/stuff_you_want_to_test.py proj/tests/test_code.py You can’t import stuff_you_want_to_test.py from the proj/tests directory by default. The more I look at the problem, the more I appreciate the simplicity of pytest-pythonpath pytest-pythonpath does one thing I really care about: Add this to a pytest.ini file at the proj level: [pytest] python_paths = src That’s it. That’s all you have to do to fix the above problem. Paths relative to the directory that pytest.ini is in. Which should be a parent or grandparent of the tests directory. I really can’t think of a simpler way for people to get around this problem. Hannah #3: Thinking in Pandas Pandas dependency hierarchy (simplified): Pandas -> NumPy -> BLAS (Basic Linear Algebra Subprograms) Languages: - - Python -> C -> Assembly df["C"] = df["A"] + df["B"] A = [ 1 4 2 0 ] B = [ 3 2 5 1 ] C = [ 1 + 3 4 + 2 2 + 5 0 + 1 ] Pandas tries to get the best performance by running operations in parallel. You might think we could speed this problem up by doing something like this: Thread 1: 1 + 3 Thread 2: 4 + 2 Thread 3: 2 + 5 Thread 4: 0 + 1 However, the GIL (Global Interpreter Lock) prevents us from achieving the performance improvement we are hoping for. Below is an example of a common threading problem and how a lock solves that problem. - Thread 1 total Thread 2 1 + 3 + 4 + 2 0 0 + 5 10 0 + 6 + 2 total += 10 0 13 total =10 0 total += 13 10 total = 13 13 Thread 1 total Thread 2 1 + 3 + 4 + 2 0 unlocked 0 + 5 10 0 unlocked + 6 + 2 total += 10 0 locked 13 total =10 0 locked 10 unlocked 10 locked total += 13 10 locked total = 13 23 unlocked As it turns out, because Python manages memory for you every object in Python would be subject to these kinds of threading issues: a = 1 # reference count = 1 b = a # reference count = 2 del(b) # reference count = 1 del(a) # reference count = 0 So, the GIL was invented to avoid this headache which only lets one thread run at a time. Certain parts of the Pandas dependency hierarchy are not subject to the GIL (simplified): Pandas -> NumPy -> BLAS (Basic Linear Algebra Subprograms) GIL -> no GIL -> hardware optimizations So we can get around the GIL in C land but what kind of optimizations does BLAS provide us with? Parallel operations inside the CPU via Vector registers A vector register is like a regular register but instead of holding one value it can hold multiple values. | 1 | 4 | 2 | 0 | + + + + | 3 | 2 | 5 | 1 | = = = = | 4 | 6 | 7 | 1 | Vector registers are only so large though, so the Dataframe is broken up into chunks and the vector operations are performed on each chunk. Michael #4: Quickle Fast. Benchmarks show it’s among the fastest serialization methods for Python. Safe. Unlike pickle, deserializing a user provided message doesn’t allow for arbitrary code execution. Flexible. Unlike msgpack or json, Quickle natively supports a wide range of Python builtin types. Versioning. Quickle supports “schema evolution”. Messages can be sent between clients with different schemas without error. Example >>> import quickle >>> data = quickle.dumps({"hello": "world"}) >>> quickle.loads(data) {'hello': 'world'} Brian #5: what(), why(), where(), explain(), more() from friendly-traceback console Do this: $ pip install friendly-friendly_traceback.install() $ python -i >>> import friendly_traceback >>> friendly_traceback.start_console() >>> Now, after an exception happens, you can ask questions about it. >>> pass = 1 Traceback (most recent call last): File "[HTML_REMOVED]", line 1 pass = 1 ^ SyntaxError: invalid syntax >>> what() SyntaxError: invalid syntax A `SyntaxError` occurs when Python cannot understand your code. >>> why() You were trying to assign a value to the Python keyword `pass`. This is not allowed. >>> where() Python could not understand the code in the file '[HTML_REMOVED]' beyond the location indicated by --> and ^. -->1: pass = 1 ^ Cool for teaching or learning. Hannah #6: Bandit Bandit is a static analysis security tool. It’s like a linter but for security issues. pip install bandit bandit -r . I prefer to run it in a git pre-commit hook: # .pre-commit-config.yaml repos: repo: https://github.com/PyCQA/bandit rev: '1.7.6' hooks: - id: bandit It finds issues like: flask_debug_true request_with_no_cert_validation You can ignore certain issues just like any other linter: assert len(foo) == 1 # nosec Extras: Brian: Meetups this week 2/3 done. NOAA Tuesday, Aberdeen this morning - “pytest Fixtures” PDX West tomorrow - Michael Presenting “Python Memory Deep Dive” Updated my training page, testandcode.com/training Feedback welcome. I really like working directly with teams and now that trainings can be virtual, a couple half days is super easy to do. Michael: PEP 634 -- Structural Pattern Matching: Specification accepted in 3.10 PyCon registration open Python Web Conf reg open Hour of code - minecraft Joke: Sent in via Michel Rogers-Vallée, Dan Bader, and Allan Mcelroy. :) PEP 8 Song Watch on YouTube By Leon Sandoy and team at Python Discord